This week’s contributing author, Sabra Sisler, is a student at Northeastern University studying neuroscience and data science.
VoCA is pleased to present this blog post in conjunction with Associate Professor of Contemporary Art History, Gloria Sutton’s Spring 2020 Honors Seminar, The Art of Visual Intelligence at Northeastern University. This interdisciplinary course combines the powers of observation (formal description, visual data) with techniques of interpretation to sharpen perceptual awareness allowing students to develop compelling analysis of visual phenomena.
Following the outbreak of COVID-19, museums have been forced to rapidly close their doors to the public for the foreseeable future. Moving programming online and creating new digital initiatives allows viewers to virtually interact with content and supplements the ways that museums have been digitizing cultural artefacts and artworks as part of their long-term conservation strategy. With this increased online activity and rush to access millions of artworks remotely, it is more important than ever to understand the ways in which our technologies are biased. Search engines are often monetarily influenced; image labelling subtly substantiates discrimination and judgement; algorithms have the power to determine what content is rendered visible and invisible; and the errant belief that technology is neutral, vindicates it all. Safiya Umoja Noble, the Co-Director of the UCLA Center for Critical Internet Inquiry, argues that “algorithms are now doing the curatorial work that human beings like librarians or teachers used to do.”1 If algorithms are the modern-day curators, those who create and design algorithms have unspeakable power to decide what artworks and artists are visible to the public.
The most significant factor impacting digital exposure of artworks is image labeling. Artworks don’t describe themselves. The creators of the project Excavating AI, a study intended to understand how image datasets are used to train AI systems state that “the project of interpreting images is a profoundly complex and relational endeavor. Images are remarkably slippery things, laden with multiple potential meanings, irresolvable questions, and contradictions.”2 Certain labels such as “tree” may be relatively innocuous, but what happens when you start labeling people? Best case scenario, labeling images of people flattens individuality by assuming identity can be determined merely by looking at an exterior. More often, it transforms subjective interpretations into concrete fact, embedding historically discriminatory and derogatory assumptions into our seemingly objective technologies. In the aforementioned Excavating AI study, a dataset commonly used to train AI systems labels an image of a woman laying on a beach towel as “kleptomaniac” and an image of a man holding a beer as “alcoholic.” To label something is to pass judgment.2 Yet, training sets for machine learning algorithms are composed entirely of labeled images. How can we say that our algorithms are unbiased when they are built on subjective assessments? The first step to mitigating bias is to provide transparent access to datasets used to create algorithms.
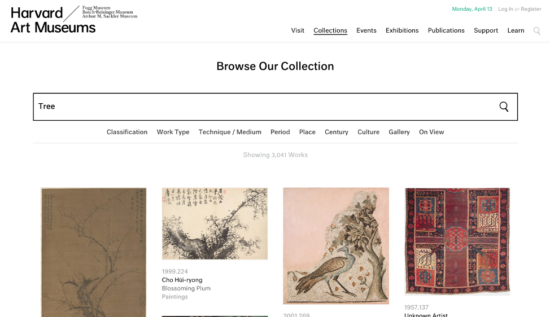
Harvard Art Museums’ Collections Webpage, 2020, https://www.harvardartmuseums.org/collections?q=Tree
To better understand how ideological issues are connected to technical protocols of database management, I spoke with Jeff Steward, the Director of Digital Infrastructure and Emerging Technology at Harvard Art Museums. Harvard Art Museums can be used as a case study to explore how public access to datasets and algorithmic transparency can mitigate bias. According to Jeff Steward, Harvard Art Museum’s mission is to “hold this great collection in trust for people. [They] are stewards of this wealth of culture and if [they] are insisting that this is something the world should hold up as important culture, [they] should do everything [they] can to make sure it is accessible not only on site in the building, but around the world.”3
Harvard Art Museums provides free digital access to all of their collections, but with a collection of over 250,000 works of art, the institution must decide how what works of art are displayed on the website. This is where protocols such as page rank—a type of algorithm used by all search engines to determine the order of results that appear after a query—come into play. Jeff Steward underscores that Harvard Art Museums “brings objects with the least number of page views and those that haven’t been viewed recently to try to circulate objects that haven’t been seen as much.”3 He says that page rank, computed daily, is influenced by factors such as presence of a collection highlight tag, date of last page view, and total page views in the past ten years. This system, although promoting exposure to lesser seen works in the collection, is still an algorithm selectively guiding the viewer’s perception of the collection. Jeff Steward believes understanding and “identifying [Harvard Art Museums’] agenda and bias up front” allows them to “[contextualize their] work and data as much as possible. [They] can provide details on the source of the data along with insight into the methodology and reasoning behind the design of the information, as well as the way [they] choose to visualize it.”4
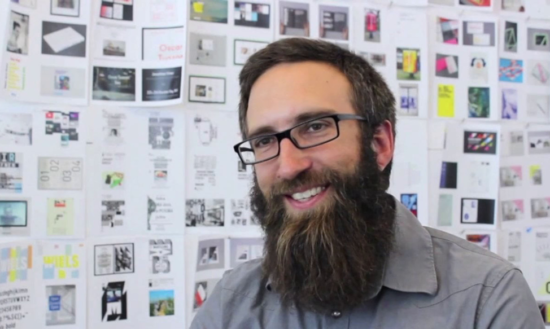
Jeff Steward, Director of Digital Infrastructure and Emerging Technology at Harvard Art Museums, https://www.harvardartmuseums.org/article/our-steward-of-technology
Harvard Art Museum also provides access to its Application Programming Interface (API), which provides the tools for a user to access all of the data tied to Harvard Art Museums’ Collections. According to Steward, “there are many reasons why the API is important to us and why it exists. They can be summed up as this: Data is at the heart of the work we do and we need an efficient and stable system for tapping into it.”5 Instead of claiming that technologies are unbiased, we should go about trying to understand where the biases exist. Other museums should use Harvard Art Museums’ digital platform as a model. Not necessarily to recreate algorithms or search results, but to emulate the transparency in which they describe the purpose of the algorithms as well as providing access to the datasets used to create such algorithms. Jeff Steward insists that “there is a variety and complexity of information that exists in the database that you can’t see on the walls or see on the main website.”3 Information is power. The transparency behind Harvard Art Museums’ digital presence allows users to understand why and how specific art works are chosen to be displayed. Providing access to the datasets used to create algorithms that impact the way we interact with digital content allows us to not only be aware of the biases, but when necessary, take measures to mitigate them.
Footnotes:
[1] Noble, S. (USC Annenberg, 2018). <https://annenberg.usc.edu/news/diversity-and-inclusion/algorithms-oppression-safiya-noble-finds-old-stereotypes-persist-new>.
[2] Crawford, K. & Paglen, T. Excavating AI: The Politics of Training Sets for Machine Learning. (2019). <https://www.excavating.ai>.
[3] Steward, J.(ed Sabra Sisler) (2020).
[4] Steward, J. The Beauty—and Complexity—of Data. (2014). <https://www.harvardartmuseums.org/article/the-beauty-and-complexity-of-data>.
[5] Harvard Art Museums API Documentation (Github).